An engineer turned program manager, our Engineering Program Manager David Lagardere is tasked with leading three of Criteo’s talented engineering teams and driving some of Criteo AI Lab’s biggest projects to success. Having spent the first fifteen years of his career in finance, David made the switch to ad tech about ten years ago and shows no signs of slowing down.
This week, David chats with us about how finance and ad tech aren’t so different, the challenges he and his team are facing now within the Criteo AI Lab, and why it’s all very exciting.
Tell us about your background and how you came to Criteo.
I started my career as an R&D engineer for a financial institution trading desk after graduating with an MSc in Computer Science. This was a fast-paced environment, requiring real-time and accurate pricing of a range of financial instruments. I found technical and business challenges so appealing that I stuck to this industry for 15 years, moving from an individual contributor role to a dev lead. I also strengthened my business skillset with an MSc in Financial Engineering.
About 10 years ago, I heard of Programmatic Advertising. From a technology perspective, it seemed that it shared many of the problems I enjoyed solving in my daily job, but everything had yet to be built. I did not jump into it right away, but familiarized myself. At some point, joining Criteo sounded like the natural move to do.
You spent the first decade of your engineering career in in Finance – how is it different from ad tech?
Although not be obvious at a first glance from a business perspective, from a technology standpoint the Financial Industry and the Digital Advertising world share a number of similarities.
For instance, financial institutions trading desks have been buying and selling a wide range of assets in a programmatic way for years. In this setup, algorithms developed by those institutions process streams of market updates in real-time, and make buying or selling decisions in a fraction of a second.
Likewise, in the digital advertising world, when a user browses a publisher’s web page, ad placements on this page are auctioned to buyers such as Criteo, whose algorithms decide whether to buy the placement and which ad to display. All this happens in real-time: every second at Criteo, we channel millions of such auctions through our Machine Learning models to show the best possible ad for a given user.
That said, modeling is another key component common to both industries: finance and ad tech both heavily rely on their ability to best predict an event or a quantity some time in the future based on past data, and require large scale, high-availability cluster computing to do so. One thing Criteo has been doing differently from the start though, is it uses Machine Learning at a very high scale to build models that make sense of the huge amount of data we deal with.
I see our area as a natural fit for engineers and researchers coming from an investment banking background.
What do you do at Criteo?
My role is Engineering Program Manager (EPM). Put simply, I drive projects to success.
More precisely, I work closely with three engineering teams, two of them being in charge of our Machine Learning Platform, which serves all our production and experimentation modeling usages. While engineers sit in the driver’s seat, building the infrastructure that fits our Product Engineering and researchers objectives, I act as a co-driver, helping anticipate those needs in collaboration with my fellow EPMs, removing or mitigating roadblocks ahead of us.
Finally, an important part of my role is to regularly share Criteo AI Lab achievements to the rest of the organization, making sure everyone is aware of what we do and why we are doing it.
What is your typical day like?
How about I describe what a typical quarter looks like? Indeed, at Criteo, we prioritize work through the OKRs framework, which means “Objective and Key results”, whereby on a quarterly basis, every R&D team sets up to 3 objectives and up to 3 milestones per objective (or Key Results). The process I drive with the engineering teams I work with is as follows:
By the last month of each quarter, we start discussing what we think next quarter priorities should be. At Criteo AI Lab, those discussions are essentially fueled by research requirements and engineering teams technical roadmaps (for instance, engineers may want get rid of a piece of technical debt, increase the robustness of an application or try out a new technology). This is a very exciting time during which we dive into research ideas to structure them into tangible deliverables. This also represents the height of EPMs activity, since it involves several iterations clarifying work needed and coordinating with other teams we may need help from to accomplish our objectives.
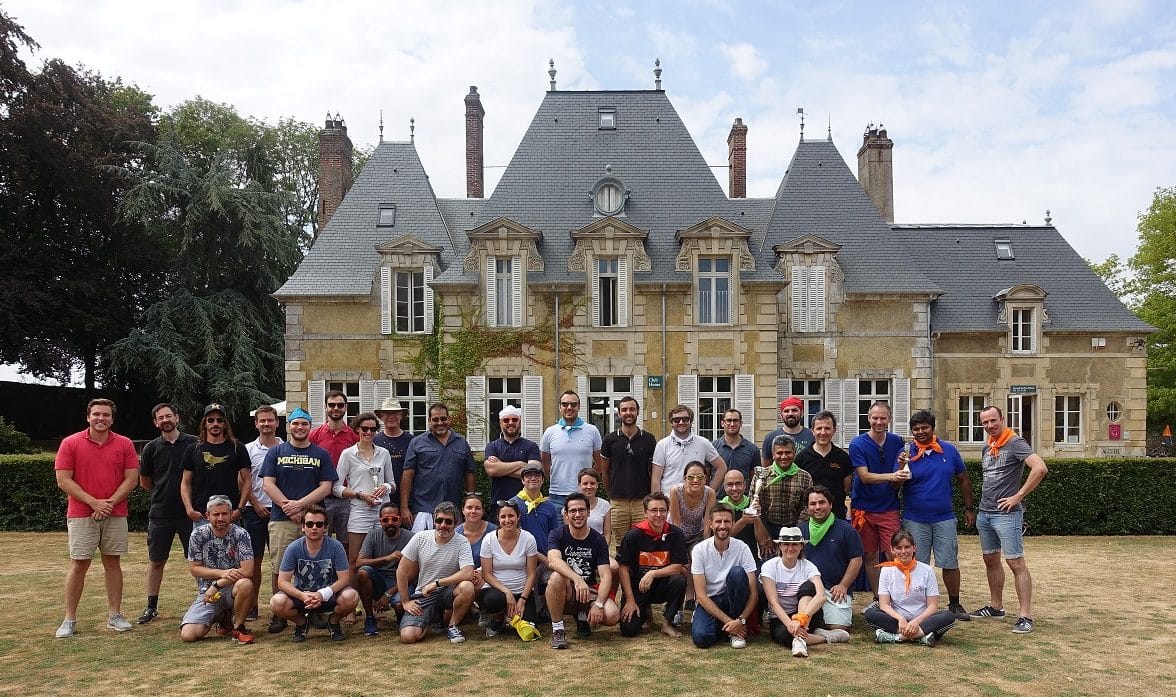
During the first two months of the quarter, there is no such thing as a typical day for EPMs. Daily work can be about following up on projects with all required parties, revising roadmaps, helping coordinating teams on production issues, working on the long term vision or putting tactical plans in place to save a project from drifting.
The one thing I do every day though, is the stand-up meeting with each of the three teams I am EPM for. On this occasion, each of us go through what they did the previous day, whether they are facing some blockers and what they are planning to do on the day. Not only this meeting allows me to stay closely connected to the team’s day to day work, but it may also reveal issues I can help with, such as clarifying/prioritizing incoming requests or issues raised by other teams.
What are you working on now?
One of my top priorities these days is to work with Engineering and Research team to upgrade our Machine Learning Platform so that open-sourced libraries such as TensorFlow can scale to Criteo data. Although backed by active communities, these libraries don’t necessarily work out of the box at our scale: we have to spend time fine-tuning and understanding potential pitfalls.
One other key project we work on is about easing our Researchers & ML Engineers everyday life with one-stop-shop Notebooks, enabling full experiment life cycle seamlessly. Finally, figuring out how to get multi-layer deep learning models to work at the latency we are used to in RTB is causing us to explore new architectures such as TPUs, IPUs, FPGA…
What’s one of your biggest challenges?
Sustained focus. Criteo AI Lab is a mix of engineering and research teams. Altogether, those teams are exploring many different directions. This yields an organizational challenge to ensure smooth collaboration. Criteo AI Lab EPMs help solving it by regularly adapting how we execute to minimize context switching between tasks and maximize each individual’s focus. At the end of the day, it all comes down to using agile practices pragmatically.
What project so far are you most proud of?
Driving the migration of our machine learning algorithms from in house implementation to Spark. I am particularly proud of this project as nearly 10+ teams across our Paris and Palo-Alto office collaborated wonderfully to make it a success.
Any advice for people getting into Machine Learning or wanting to apply to Criteo ?
Get ready to express your drive and creativity! At Criteo, you can have an impact in so many ways beside doing your job: you are empowered to come up with new product ideas, new technologies, new project management techniques… and go all the way to testing them live! It is basically all up to you.